ABSTRACT: Ion suppression is a major problem in mass spectrometry (MS)-based metabolomics; it can dramatically decrease measurement accuracy, precision, and sensitivity. Here we report a method, the IROA TruQuant Workflow, that uses a stable isotope-labeled internal standard (IROA-IS) library plus companion algorithms to: 1) measure and correct for ion suppression, and 2) perform Dual MSTUS normalization of MS metabolomic data. We evaluate the method across ion chromatography (IC), hydrophilic interaction liquid chromatography (HILIC), and reversed-phase liquid chromatography (RPLC)-MS systems in both positive and negative ionization modes, with clean and unclean ion sources, and across different biological matrices. Across the broad range of conditions tested, all detected metabolites exhibit ion suppression ranging from 1% to >90% and coefficients of variation ranging from 1% to 20%, but the Workflow and companion algorithms are highly effective at nulling out that suppression and error. To demonstrate a routine application of the Workflow, we employ the Workflow to study ovarian cancer cell response to the enzyme-drug L-asparaginase (ASNase). The IROA-normalized data reveal significant alterations in peptide metabolism, which have not been reported previously. Overall, the Workflow corrects ion suppression across diverse analytical conditions and produces robust normalization of non-targeted metabolomic data.
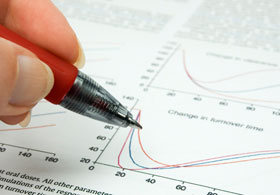
Katrice A. Lippa, Juan J. Aristizabal-Henao, Richard D. Beger, John A. Bowden, Corey Broeckling, Chris Beecher, W. Clay Davis, Warwick B. Dunn, Roberto Flores, Royston Goodacre, Gonçalo J. Gouveia, Amy C. Harms, Thomas Hartung, Christina M. Jones, Matthew R. Lewis, Ioanna Ntai, Andrew J. Percy, Dan Raftery, Tracey B. Schock, Jinchun Sun, Georgios Theodoridis, Fariba Tayyari, Federico Torta, Candice Z. Ulmer, Ian Wilson & Baljit K. Ubhi
We are proud supporters of mQACC and honored to contribute to this Review Article to improve metabolomics data quality.
Introduction
The metabolomics quality assurance and quality control consortium (mQACC) is enabling the identification, development, prioritization, and promotion of suitable reference materials (RMs) to be used in quality assurance (QA) and quality control (QC) for untargeted metabolomics research.
Objectives
This review aims to highlight current RMs, and methodologies used within untargeted metabolomics and lipidomics communities to ensure standardization of results obtained from data analysis, interpretation and cross-study, and cross-laboratory comparisons. The essence of the aims is also applicable to other ‘omics areas that generate high dimensional data.
Results
The potential for game-changing biochemical discoveries through mass spectrometry-based (MS) untargeted metabolomics and lipidomics are predicated on the evolution of more confident qualitative (and eventually quantitative) results from research laboratories. RMs are thus critical QC tools to be able to assure standardization, comparability, repeatability and reproducibility for untargeted data analysis, interpretation, to compare data within and across studies and across multiple laboratories. Standard operating procedures (SOPs) that promote, describe and exemplify the use of RMs will also improve QC for the metabolomics and lipidomics communities.
Conclusions
The application of RMs described in this review may significantly improve data quality to support metabolomics and lipidomics research. The continued development and deployment of new RMs, together with interlaboratory studies and educational outreach and training, will further promote sound QA practices in the community.
Chris Beecher, Felice A. de Jong, IROA Technologies
DOI: 10.13140/RG.2.2.28112.74245
ABSTRACT: The lack of reproducible data due to matrix effects in untargeted metabolomics has been an on-going major concern. Ion suppression, interference from coeluted chemical components (sample matrix, solvent or LC-MS system components), is often observed as the loss of analyte ionization response and negatively affects precision, accuracy, and reproducibility (over- or underestimation of analyte levels). The optimization and validation of quantitative LC-MS methods relies on the assessment and correction of ion suppression. We conducted a simple experimental to understand the level of suppression of compounds in a common human plasma sample that had been serially diluted. Once the impact of ion suppression was assessed and quantified, we developed a workflow to minimize variability and to correct the ion suppressed data which then could be effectively normalized to achieve reproducible measurements. Why work up bad data?
Jordan Carey1, Thanh Nguyen1, Jennifer Korchak1, Christopher Beecher2, Felice de Jong2, and Amy L. Lane1
1 Chemistry Department, University of North Florida, Jacksonville, FL 32224, USA; 2 IROA Technologies, Ann Arbor, MI 48105, USA
Metabolites 2019, 9(9), 181; https://doi.org/10.3390/metabo9090181
ABSTRACT: Actinomycetes are powerhouses of natural product biosynthesis. Full realization of this biosynthetic potential requires approaches for recognizing novel metabolites and determining mediators of metabolite production. Herein, we develop an isotopic ratio outlier analysis (IROA) ultra-high performance liquid chromatography-mass spectrometry (UHPLC/MS) global metabolomics strategy for actinomycetes that facilitates recognition of novel metabolites and evaluation of production mediators. We demonstrate this approach by determining impacts of the iron chelator 2,2′-bipyridyl on the Nocardiopsis dassonvillei metabolome. Experimental and control cultures produced metabolites with isotopic carbon signatures that were distinct from corresponding “standard” culture metabolites, which were used as internal standards for LC/MS. This provided an isotopic MS peak pair for each metabolite, which revealed the number of carbon atoms and relative concentrations of metabolites and distinguished biosynthetic products from artifacts. Principal component analysis (PCA) and random forest (RF) differentiated bipyridyl-treated samples from controls. RF mean decrease accuracy (MDA) values supported perturbation of metabolites from multiple amino acid pathways and novel natural products. Evaluation of bipyridyl impacts on the nocazine/XR334 diketopiperazine (DKP) pathway revealed upregulation of amino acid precursors and downregulation of late stage intermediates and products. These results establish IROA as a tool in the actinomycete natural product chemistry arsenal and support broad metabolic consequences of bipyridyl.
Roberto Mendez1, Maria del Carmen Piqueras2, Alexander Raskind3, Felice A. de Jong3, Chris Beecher3,4, Sanjoy K. Bhattacharya2,5, and Santanu Banerjee6,7,8
1 Department of Surgery, Miller School of Medicine, University of Miami, Miami, FL, USA; 2 Bascon Palmer Eye Institute, University of Miami Miller School of Medicine, Miami, FL, USA; 3 IROA Technologies LLC, Bolton, MA, USA; 4 SECIM, University of Florida, Gainesville, FL, USA; 5 Miami Integrative Metabolomic Research Center, University of Miami, Miami, FL, USA; 6 Department of Surgery, Miller School of Medicine, University of Miami, Miami, FL, USA; 7 Miami Integrative Metabolomic Research Center, University of Miami, Miami, FL, USA; 8 Sylvester Comprehensive Cancer Center, University of Miami, Miami, FL, USA.
Published in Methods in Molecular Biology 2019;https://doi.org/10.1007/978-1-4939-9488-5
Various research strategies involving biomarker discovery and mechanistic studies in system biology depend on reproducible and reliable quantification of all metabolites from tissue(s) of interest. Contemporary analytical methods rely on mass spectrometry-based targeted and/or untargeted metabolomics platforms.
ABSTRACT: The robustness of these analyses depends on the cleanliness of the samples, accuracy of the database, resolution of the instrument, and, the most variable of the list, the personal preferences of the researcher and the instrument operator. In this chapter, we introduce a simple method to prepare murine liver samples and carry it through the Isotope Ratio Outlier Analysis (IROA®) pipeline. This pipeline encompasses sample preparation, LC-MS-based peak acquisition, proprietary software-based library creation, normalization, and quantification of metabolites. IROA® offers a unique platform to create and normalize a local library and account for run-to-run variability over years of acquisition using the internal standards (IROA®-IS) and long-term reference standards (IROA®-LTRS).
Chris Vlahakis 1, Jan Hazebroek 1, Chris Beecher 2, Felice de Jong 2
1 DuPont Pioneer, 8325 NW 62nd, Avenue, Johnston, IA, 50131-1004, USA; 2 IROA Technologies, 184 Century Mill Road, Bolton, MA, 01740, USA
https://doi.org/10.1016/j.phytochem.2019.05.006
ABSTRACT: We evaluated Isotope Ratio Outlier Analysis (IROA) as a metabolome-wide internal standard approach to improve the quality of LC/MS data collected from a large-scale greenhouse experiment designed to metric the ability of metabolomics to model quantitatively nitrogen treatments. We further looked at how IROA would be incorporated into a metabolomics workflow. For this we compared IROA processed data with that generated without the benefit of metabolome-wide internal standards using our current tool, Genedata Expressionist, from the same raw LC/MS data files. In our experiment, 367 maize plants were grown from kernel in a greenhouse under controlled conditions. Plants were treated from germination on with varying concentrations of nutrient nitrogen as one (treatment) variable. A second variable was the presence of one of two transgenes. Metabolomics analysis of leaves was performed by LC/MS positive and negative electrospray ionization modes, and raw data were processed with both our routine and IROA protocols. IROA data analysis detected 184 metabolites in each ionization mode. Analysis without IROA yielded 281 metabolites in positive ionization mode and 172 in negative ionization mode. Data from both protocols were normalized for sample dry weight, location in the greenhouse, extraction batch, sample run order, and internal standard. Normalized results were subjected to partial least squares (PLS) analysis to model the relationship between the metabolome and nitrogen treatment. Without IROA, regression coefficients of 0.819 and 0.849 for positive and negative modes, respectively were achieved. The IROA protocol improved on the values, yielding regression coefficients of 0.876 and 0.879 for positive and negative modes, respectively. In addition, IROA corrected for detector saturation for several high abundant peaks. Our experiment demonstrates that incorporating IROA into an LC/MS metabolomics experiment improves data quality and facilitates more precise modeling of a biological response.
Yunping Qiu 1 ID , Robyn D. Moir 2, Ian M.Willis 2, Suresh Seethapathy 3, Robert C. Biniakewitz 3 and Irwin J. Kurland1
1 Stable Isotope and Metabolomics Core Facility, Diabetes Center, Department of Medicine, Albert Einstein College of Medicine, Bronx, NY 10461, USA; Yunping.qiu@einstein.yu.edu; 2 Department of Biochemistry, Albert Einstein College of Medicine, Bronx, NY 10461, USA; robyn.moir@einstein.yu.edu (R.D.M.); ian.willis@einstein.yu.edu (I.M.W.); 3 Thermo Fisher Scientific, Somerset, NJ 08873, USA; suresh.seethapathy@thermofisher.com (S.S.); robert.biniakewitz@thermofisher.com (R.C.B.)
Metabolites 2018, 8, 9; doi:10.3390/metabo8010009
ABSTRACT: Identifying non-annotated peaks may have a significant impact on the understanding of biological systems. In silico methodologies have focused on ESI LC/MS/MS for identifying non-annotated MS peaks. In this study, we employed in silico methodology to develop an Isotopic Ratio Outlier Analysis (IROA) workflow using enhanced mass spectrometric data acquired with the ultra-high resolution GC-Orbitrap/MS to determine the identity of non-annotated metabolites. The higher resolution of the GC-Orbitrap/MS, together with its wide dynamic range, resulted in more IROA peak pairs detected, and increased reliability of chemical formulae generation (CFG). IROA uses two different 13C-enriched carbon sources (randomized 95% 12C and 95% 13C) to produce mirror image isotopologue pairs, whose mass difference reveals the carbon chain length (n), which aids in the identification of endogenous metabolites. Accurate m/z, n, and derivatization information are obtained from our GC/MS workflow for unknown metabolite identification, and aids in silico methodologies for identifying isomeric and non-annotated metabolites. We were able to mine more mass spectral information using the same Saccharomyces cerevisiae growth protocol (Qiu et al. Anal. Chem 2016) with the ultra-high resolution GC-Orbitrap/MS, using 10% ammonia in methane as the CI reagent gas. We identified 244 IROA peaks pairs, which significantly increased IROA detection capability compared with our previous report (126 IROA peak pairs using a GC-TOF/MS machine). For 55 selected metabolites identified from matched IROA CI and EI spectra, using the GC-Orbitrap/MS vs. GC-TOF/MS, the average mass deviation for GC-Orbitrap/MS was 1.48 ppm, however, the average mass deviation was 32.2 ppm for the GC-TOF/MS machine. In summary, the higher resolution and wider dynamic range of the GC-Orbitrap/MS enabled more accurate CFG, and the coupling of accurate mass GC/MS IROA methodology with in silico fragmentation has great potential in unknown metabolite dentification, with applications for characterizing model organism networks.
*Co-first authors.
Mohammad Atikur Rahman 1*, Masum Akond 1*, Md Ali Babar1, Chris Beecher 2,3, John Erickson 1, Kayla Thomason 1, Felice A de Jong 3, Richard Esten Mason 4
1 Agronomy Department, IFAS, University of Florida, Gainesville, FL, USA; 2 Southeast Center for Integrated Metabolomics (SECIM), University of Florida, Gainesville, FL, USA; 3 IROA Technologies LLC, Ann Arbor, MI, USA, 4 Crop, Soil and Environmental Sciences, University of Arkansas, Fayetteville, AR, USA
American Journal of Plant Sciences 2017,8:3024-3061. doi:10.4236/ajps.2017.812205.
ABSTRACT: Drought stress at the reproductive stage causes severe damage to productivity of wheat. However, little is known about the metabolites associated with drought tolerance. The objectives of this study were to elucidate changes in metabolite levels in wheat under drought, and to identify potential metabolites associated with drought stress through untargeted metabolomic profiling using a liquid chromatography-high resolution mass spectrometry (LC-HRMS)-based technique called Isotopic Ratio Outlier Analysis. Metabolomic analysis was performed on flag leaves of drought-stressed and control (well-watered) plants after 18 days of post-anthesis drought stress at three-hour intervals over a 24-hour period. Out of 723 peaks detected in leaves, 221 were identified as known metabolites. Sixty known metabolites were identified as important metabolites by 3 different methods, PLS-DA, RF and SAM. The most pronounced accumulation due to drought stress was demonstrated by tryptophan, proline, pipecolate and linamarin, whereas the most pronounced decrease was demonstrated by serine, trehalose, N-acetyl-glutamic acid, DIBOA-glucoside etc. Three different patterns of metabolite accumulation were observed over 24-hour period. The increased accumulated metabolites remained higher during all 8 time points in drought stressed leaves. On the contrary, metabolites that showed decreased level remained significantly lower during all or the most time points. However, the levels of some decreased metabolites were lower during the day, but higher during night in drought stressed leaves. Both univariate and multivariate analyses predicted thatN-acetyl-glutamic acid, proline, pipecolate, linamarin, tryptophan, and DIBOA-glucoside could be potential metabolite biomarkers, and their levels could serve as indicators of drought tolerance in wheat.
Mark R. Viant 1,2, Irwin J. Kurland 3, Martin R. Jones 1, Warwick B. Dunn 1,2
1 School of Biosciences, University of Birmingham, Edgbaston, Birmingham B15 2TT, UK; 2 Phenome Centre Birmingham, University of Birmingham, Edgbaston, Birmingham B15 2TT, UK,3 Albert Einstein College of Medicine, 1300 Morris Park Avenue, Bronx, NY 10461, USA
Current Opinion in Chemical Biology 2017, 36:64–69
ABSTRACT: The metabolome is critical for discovering the maximum amount of biochemical knowledge from metabolomics datasets. Yet no exhaustive experimental characterisation of any organismal metabolome has been reported to date, dramatically contrasting with the genome sequencing of thousands of plants, animals and microbes. Here, we review the status of metabolome annotation and describe advances in the analytical methodologies being applied. In part through new international coordination, we conclude that we are now entering a new era of metabolome annotation.
Yunping Qiu 1, Robin Moir 1, Ian M. Willis 1, Chris Beecher 2, Yu-Hsuan Tsai 1, Timothy J. Garrett 3, Richard A. Yost 3, Irwin Jack Kurland 1
1 Albert Einstein College of Medicine, Bronx, NY, USA, 2 IROA Technologies, Ann Arbor, MI, USA, 3 University of Florida, Gainesville, FL, USA
First report using IROA technology in combination with accurate mass GC/TOF-MS, used to examine the S. cerevisiae metabolome. An accuratemass CI IROA library containing 126 metabolites with retention times based on the Fiehn protocol was established. The combination of CI and EI IROA protocols identifies co-eluting metabolites and differentiates metabolite spectra from artifacts, and “known unknown” metabolites were easily separated, with the number of carbons identified from the IROA patterns.
Analytical Chemistry 88:5. doi. 10.1021/acs.analchem.5b04263, January 2016
ABSTRACT: Isotopic Ratio Outlier Analysis (IROA) is a 13C metabolomics profiling method that eliminates sample-to-sample variance, discriminates against noise and artifacts, and improves identification of compounds, previously done with accurate mass LC/MS. This is the first report using IROA technology in combination with accurate mass GC-TOFMS, here used to examine the S. cerevisiae metabolome. S. cerevisiae was grown in YNB media, containing randomized 95% 13C, or 5%13C glucose as the single carbon source, in order that the isotopomer pattern of all metabolites would mirror the labeled glucose. When these IROA experiments are combined, the abundance of the heavy isotopologues in the 5%13C extracts, or light isotopologues in the 95%13C extracts, follows the binomial distribution, showing mirrored peak pairs for the molecular ion. The mass difference between the 12C monoisotopic and the 13C monoisotopic equals the number of carbons in the molecules. The IROA-GC/MS protocol developed, using both Chemical and Electron Ionization, extends the information acquired from the isotopic peak patterns for formulae generation, a process that can be formulated as an algorithm, in which the number of carbons, as well as the number of methoximations and silylations, are used as search constraints. In Electron Impact (EI/IROA) spectra, the artifactual peaks are identified and easily removed, which has the potential to generate “clean” EI libraries. The combination of Chemical Ionization (CI) IROA and EI IROA affords a metabolite identification procedure that enables the identification of co-eluting metabolites, and allowed us to characterize 126 metabolites in the current study.
Chaevien S. Clendinen 1,2, Gregory S. Stupp 3, Ramadan Ajredini 1,2, Brittany Lee-McMullen 1,2, Chris Beecher 1,2,4 and Arthur S. Edison 1,2
1 Southeast Center for Integrated Metabolomics, University of Florida, Gainesville, FL, USA, 2 Department of Biochemistry and Molecular Biology, University of Florida, Gainesville, FL, USA, 3 The Scripps Research Institute, La Jolla, CA, USA, 4IROA Technologies, Ann Arbor, MI, USA
Frontiers in Plant Science 6:611. doi. 10.3389/fpls.2015.00611, August 2015
ABSTRACT: Compound identification is a major bottleneck in metabolomics studies. In nuclear magnetic resonance (NMR) investigations, resonance overlap often hinders unambiguous database matching or de novo compound identification. In liquid chromatography mass spectrometry (LC-MS), discriminating between biological signals and background artifacts and reliable determination of molecular formulae are not always straightforward.
We have designed and implemented several NMR and LC-MS approaches that utilize 13C, either enriched or at natural abundance, in metabolomics applications. For LC-MS applications, we describe a technique called isotopic ratio outlier analysis (IROA), which utilizes samples that are isotopically labeled with 5% (test) and 95% (control) 13C. This labeling strategy leads to characteristic isotopic patterns that allow the differentiation of biological signals from artifacts and yield the exact number of carbons, significantly reducing possible molecular formulae. The relative abundance between the test and control samples for every IROA feature can be determined simply by integrating the peaks that arise from the 5 and 95% channels. For NMR applications, we describe two 13Cbased approaches. For samples at natural abundance, we have developed a workflow to obtain 13C–13C and 13C–1H statistical correlations using 1D 13C and 1H NMR spectra.
For samples that can be isotopically labeled, we describe another NMR approach to obtain direct 13C–13C spectroscopic correlations. These methods both provide extensive information about the carbon framework of compounds in the mixture for either database matching or de novo compound identification. We also discuss strategies in which 13C NMR can be used to identify unknown compounds from IROA experiments. By combining technologies with the same samples, we can identify important biomarkers and corresponding metabolites of interest.
Arthur S. Edison1* , Chaevien S. Clendinen*, Ramadan Ajredini*, Chris Beecher*,†, Francesca V. Ponce* and Gregory S. Stupp‡
*Department of Biochemistry and Molecular Biology and Southeast Center for Integrated Metabolomics, University of Florida, Gainesville, FL 32610-0245, USA; †IROA Technologies, Ann Arbor, MI, USA; ‡The Scripps Research Institute, Department of Molecular and Experimental Medicine, 10550 North Torrey Pines Road, La Jolla, CA 92037, USA
Integrative and Comparative Biology DOI:10.1093/ICH/ICV077. Publication Date (Web): July 2, 2015
ABSTRACT: This review provides an overview of two complementary approaches to identify biologically active compounds for studies in chemical ecology. The first is activity-guided fractionation and the second is metabolomics, particularly focusing on a new liquid chromatography-mass spectrometry-based method called isotopic ratio outlier analysis. To illustrate examples using these approaches, we review recent experiments using Caenorhabditis elegans and related free-living nematodes.
Gregory S. Stupp†, Chaevien S. Clendinen†, Ramadan Ajredini†, Mark A. Szewc‡ , Timothy Garrett § , Robert F. Menger , Richard A. Yost, , Chris Beecher , and Arthur S. Edison†
†Department of Biochemistry and Molecular Biology,University of Florida, Gainesville, FL; ‡ Thermo Fisher Scientific, Somerset, NJ; §Department of Pathology, Immunology, and Laboratory Medicine; Southeast Center for Integrated Metabolomics; Department of Chemistry,University of Florida, Gainesville, FL; IROA Technologies, Ann Arbor, MI
Anal. Chem. DOI: 10.1021/ac4025413. Publication Date (Web): November 25, 2013
ABSTRACT: We demonstrate the global metabolic analysis of Caenorhabditis elegans stress responses using a mass-spectrometry-based technique called isotopic ratio outlier analysis (IROA). In an IROA protocol, control and experimental samples are isotopically labeled with 95 and 5% 13C, and the two sample populations are mixed together for uniform extraction, sample preparation, and LC-MS analysis. This labeling strategy provides several advantages over conventional approaches: (1) compounds arising from biosynthesis are easily distinguished from artifacts, (2) errors from sample extraction and preparation are minimized because the control and experiment are combined into a single sample, (3) measurement of both the molecular weight and the exact number of carbon atoms in each molecule provides extremely accurate molecular formulas, and (4) relative concentrations of all metabolites are easily determined. A heat-shock perturbation was conducted on C. elegans to demonstrate this approach. We identified many compounds that significantly changed upon heat shock, including several from the purine metabolism pathway. The metabolomic response information by IROA may be interpreted in the context of a wealth of genetic and proteomic information available for C. elegans. Furthermore, the IROA protocol can be applied to any organism that can be isotopically labeled, making it a powerful new tool in a global metabolomics pipeline.
Paul Fogel†, Douglas M. Hawkins‡, Chris Beecher , George Luta || and S. Stanley Young*
† Consultant, Paris; ‡ School of Statistics, University of Minnesota, Minneapolis, MN; Department of Biostatistics, Bioinformatics, and Biomathematics, Georgetown University Medical Center, Washington DC; IROA Technologies, Ann Arbor, MI; *National Institute of Statistical Sciences, Research Triangle Park, NC
The American Statistician, Volume 67, Issue 4, 2013
ABSTRACT: In statistical practice, rectangular tables of numeric data are commonplace, and are often analyzed using dimension-reduced methods like the singular value decomposition and its close cousin, principal component analysis (PCA). This analysis produces score and loading matrices representing the rows and columns of the original table and these matrices may be used for both prediction purposes and to gain structural understanding of the data. In some tables, the data entries are necessarily nonnegative (apart, perhaps, from some small random noise), and so the matrix factors meant to represent them should arguably also contain nonnegative elements. This thinking, and the desire for parsimony, underlies such techniques as rotating factors in a search for “simple structure.” These attempts to transform score or loading matrices of mixed sign into nonnegative matrix factorization, or NMF, is an attractive alternative. Rather than attempt to transform a loading or score matrix of mixed signs into one with only nonnegative elements, it directly seeks matrix factors containing only nonegative elements. The resulting factorization often leads to substantial improvements in interpretability of the factors. We illustrate this potential by synthetic examples anda real dataset. The questions of exactly when NMF is effective is not fully resolved, but some indicators of its domain of success are given. It is pointed out that the NMF factors can be used in much of the same way as those coming from PCA for such tasks as ordination, clustering, and prediction. Supplementary materials for this article are available on line.
Felice A. de Jong and Chris Beecher, IROA Technologies, Ann Arbor, MI
Bioanalysis, Volume 4, Issue 18, 2012
ABSTRACT: Metabolomics or biochemical profiling is a fast emerging science; however, there are still many associated bottlenecks to overcome before measurements will be considered robust. Advances in MS resolution and sensitivity, ultra-pressure LC MS, ESI, and isotopic approaches such as flux analysis and stable-isotope dilution, have made it easier to quantitate biochemicals. The digitization of mass spectrometers has simplified informatic aspects. However, issues of analytical variability, ion suppression and metabolite identification still plague metabolomics investigators. These hurdles need to be overcome for accurate metabolite quantitation not only for in vitro systems, but for complex matrices such as biofluids and tissues, before it is possible to routinely identify biomarkers that are associated with the early prediction and diagnosis of diseases. In this report, we describe a novel isotopic-labeling method that uses the creation of distinct biochemical signatures to eliminate current bottlenecks and enable accurate metabolic profiling.